From Boolean to Probabilistic Boolean Networks as Models of Genetic Regulatory Networks
摘要:
Mathematical and computational modeling of genetic regulatory networks promises to uncover the fundamental principles governing biological systems in an integrative and holistic manner. It also paves the way toward the development of systematic approaches for effective therapeutic intervention in disease. The central theme in this paper is the Boolean formalism as a building block for modeling complex, large-scale, and dynamical networks of genetic interactions. We discuss the goals of modeling genetic networks as well as the data requirements. The Boolean formalism is justified from several points of view. We then introduce Boolean networks and discuss their relationships to nonlinear digital filters. The role of Boolean networks in understanding cell differentiation and cellular functional states is discussed. The inference of Boolean networks from real gene expression data is considered from the viewpoints of computational learning theory and nonlinear signal processing, touching on computational complexity of learning and robustness. Then, a discussion of the need to handle uncertainty in a probabilistic framework is presented, leading to an introduction of probabilistic Boolean networks and their relationships to Markov chains. Methods for quantifying the influence of genes on other genes are presented. The general question of the potential effect of individual genes on the global dynamical network behavior is considered using stochastic perturbation analysis. This discussion then leads into the problem of target identification for therapeutic intervention via the development of several computational tools based on first-passage times in Markov chains. Examples from biology are presented throughout the paper.
展开
关键词:
Bibliography, Theoretical or Mathematical/ Boolean functions computational complexity genetics Markov processes physiological models probability/ cellular functional states cell differentiation computational complexity target identification problem stochastic perturbation analysis therapeutic intervention nonlinear digital filters genes influence quantification learning robustness first-passage times disease/ C1290L Systems theory applications in biology and medicine C1140J Markov processes C1220 Simulation, modelling and identification C4240C Computational complexity
DOI:
10.1109/JPROC.2002.804686
被引量:
年份:
2002
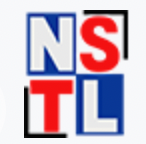


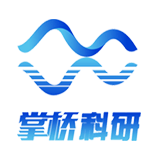





























通过文献互助平台发起求助,成功后即可免费获取论文全文。
相似文献
参考文献
引证文献
辅助模式
引用
文献可以批量引用啦~
欢迎点我试用!