Boosting for tumor classification with gene expression data
摘要:
MOTIVATION: Microarray experiments generate large datasets with expression values for thousands of genes but not more than a few dozens of samples. Accurate supervised classification of tissue samples in such high-dimensional problems is difficult but often crucial for successful diagnosis and treatment. A promising way to meet this challenge is by using boosting in conjunction with decision trees. RESULTS: We demonstrate that the generic boosting algorithm needs some modification to become an accurate classifier in the context of gene expression data. In particular, we present a feature preselection method, a more robust boosting procedure and a new approach for multi-categorical problems. This allows for slight to drastic increase in performance and yields competitive results on several publicly available datasets. AVAILABILITY: Software for the modified boosting algorithms as well as for decision trees is available for free in R at http://stat.ethz.ch/~dettling/boosting.html.
展开
关键词:
Algorithms Artificial Intelligence Gene Expression Profiling Genetic Screening 算法 人工智能 遗传筛选 肿瘤蛋白质类 肿瘤 寡核苷酸序列分析
DOI:
10.1093/bioinformatics/bth447
被引量:
年份:
2004

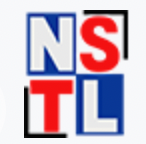
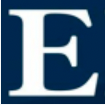


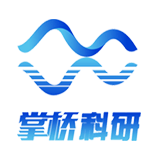




























































通过文献互助平台发起求助,成功后即可免费获取论文全文。
相似文献
参考文献
引证文献
辅助模式
引用
文献可以批量引用啦~
欢迎点我试用!