Automated Learning of Probabilistic Assumptions for Compositional Reasoning
摘要:
Probabilistic verification techniques have been applied to the formal modelling and analysis of a wide range of systems, from commu- nication protocols such as Bluetooth, to nanoscale computing devices, to biological cellular processes. In order to tackle the inherent challenge of scalability, compositional approaches to verification are sorely needed. An example is assume-guarantee reasoning, where each component of a system is analysed independently, using assumptions about the other components that it interacts with. We discuss recent developments in the area of automated compositional verification techniques for probabilistic systems. In particular, we describe techniques to automatically generate probabilistic assumptions that can be used as the basis for compositional reasoning. We do so using algorithmic learning techniques, which have already proved to be successful for the generation of assumptions for compositional verification of non-probabilistic systems. We also present recent improvements and extensions to this work and survey some of the promising potential directions for further research in this area.
展开
DOI:
10.1007/978-3-642-19811-3_2
被引量:
年份:
2011



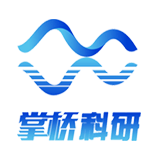





















































通过文献互助平台发起求助,成功后即可免费获取论文全文。
相似文献
参考文献
引证文献
辅助模式
引用
文献可以批量引用啦~
欢迎点我试用!